At Waymo, we have been at the forefront of AI and ML in autonomous driving for over 15 years, and are continuously contributing to advancing research in the field. Today, we are sharing our latest research paper on an End-to-End Multimodal Model for Autonomous Driving (EMMA).
Powered by Gemini, a multimodal large language model developed by Google, EMMA employs a unified, end-to-end trained model to generate future trajectories for autonomous vehicles directly from sensor data. Trained and fine-tuned specifically for autonomous driving, EMMA leverages Gemini’s extensive world knowledge to better understand complex scenarios on the road.
Our research demonstrates how multimodal models, such as Gemini, can be applied to autonomous driving and explores pros and cons of the pure end-to-end approach. It highlights the benefit of incorporating multimodal world knowledge, even when the model is fine-tuned for autonomous driving tasks that require good spatial understanding and reasoning skills. Notably, EMMA demonstrates positive task transfer across several key autonomous driving tasks: training it jointly on planner trajectory prediction, object detection, and road graph understanding leads to improved performance compared to training individual models for each task. This suggests a promising avenue of future research, where even more core autonomous driving tasks could be combined in a similar, scaled-up setup.
“EMMA is research that demonstrates the power and relevance of multimodal models for autonomous driving,” said Waymo VP and Head of Research Drago Anguelov. “We are excited to continue exploring how multimodal methods and components can contribute towards building an even more generalizable and adaptable driving stack.”
Introducing EMMA
EMMA reflects efforts in the broader AI research landscape to integrate large-scale multimodal learning models and techniques into more domains. Building on top of Gemini and leveraging its capabilities, we created a model tailored for autonomous driving tasks such as motion planning and 3D object detection.
Key aspects of this research include:
- End-to-End Learning: EMMA processes raw camera inputs and textual data to generate various driving outputs including planner trajectories, perception objects, and road graph elements.
- Unified Language Space: EMMA maximizes Gemini’s world knowledge by representing non-sensor inputs and outputs as natural language text.
- Chain-of-Thought Reasoning: EMMA uses chain-of-thought reasoning to enhance its decision-making process, improving end-to-end planning performance by 6.7% and providing interpretable rationale for its driving decisions.
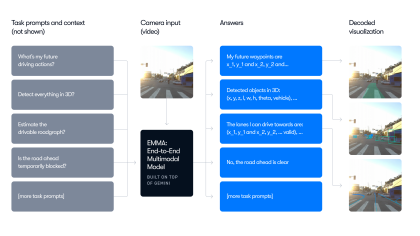
Co-training EMMA on multiple tasks
EMMA achieves state-of-the-art or competitive results on multiple autonomous driving tasks on public and internal benchmarks, including end-to-end planning trajectory prediction, camera-primary 3D object detection, road graph estimation, and scene understanding.
One of the most promising aspects of EMMA is its ability to improve through co-training. A single co-trained EMMA can jointly produce the outputs for multiple tasks, while matching or even surpassing the performance of individually trained models, highlighting its potential as a generalist model for many autonomous driving applications.
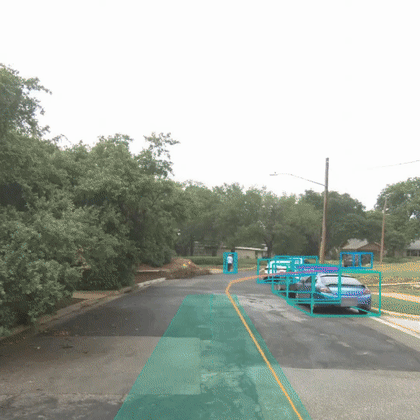
EMMA navigating in dense urban traffic, and yielding for a dog on the road, even though it was not trained to detect this object category. The yellow line represents roadgraph estimation, and the green stripe shows future planning trajectory.
While EMMA shows great promise, we recognize several of its challenges. EMMA’s current limitations in processing long-term video sequences restricts its ability to reason about real-time driving scenarios — long-term memory would be crucial in enabling EMMA to anticipate and respond in complex evolving situations. Other key challenges to ensure safe driving behavior include EMMA not leveraging LiDAR and radar inputs, which requires the fusion of more sophisticated 3D sensing encoders, the challenge of efficient simulation methods for evaluation, the need for optimized model inference time, and verification of intermediate decision-making steps.
The Future of AI at Waymo
Despite the above challenges of EMMA as a standalone model for driving, this research work highlights the benefits of enhancing AV system performance and generalizability with multimodal techniques.
The significance of this research extends beyond autonomous vehicles. By applying cutting-edge AI technologies to real-world tasks, we are expanding AI’s capabilities in complex, dynamic environments. This progress could lead to AI assisting in other critical areas requiring rapid, informed decision-making based on multiple inputs in unpredictable situations. As we explore the potential of multimodal large language models in autonomous driving, we remain committed to enhancing road safety and accessibility. This work opens exciting avenues for AI to navigate and reason about complex real world environments more effectively in the future.
If you’re passionate about solving some of the most interesting and impactful challenges in AI, we invite you to explore career opportunities with us and help shape the future of transportation. For those interested in diving deeper into the technical details of this research, you can read our full paper here.
https://waymo.com/blog/2024/10/introducing-emma/