AI is transforming industries, enterprises, and consumer experiences in new ways. Generative AI models are moving towards reasoning, agentic AI is enabling new outcome-oriented workflows and physical AI is enabling endpoints like cameras, robots, drones, and cars to make decisions and interact in real time.
The common glue between all these use cases is the need for pervasive, reliable, secure, and super-fast connectivity.
Telecommunication networks must prepare for this new kind of AI traffic, which can come directly through the fronthaul wireless access network or backhauled from the public or private cloud as a completely standalone AI inferencing traffic generated by enterprise applications.
Local wireless infrastructure offers an ideal place to process AI inferencing. This is where a new approach to telco networks, AI radio access network (AI-RAN), stands out.
Traditional CPU or ASIC-based RAN systems are designed only for RAN use and cannot process AI traffic today. AI-RAN enables a common GPU-based infrastructure that can run both wireless and AI workloads concurrently, turning networks from single-purpose to multi-purpose infrastructures and turning sites from cost-centers to revenue sources.
With a strategic investment in the right kind of technology, telcos can leap forward to become the AI grid that facilitates the creation, distribution, and consumption of AI across industries, consumers, and enterprises. This moment in time presents a massive opportunity for telcos to build a fabric for AI training (creation) and AI inferencing (distribution) by repurposing their central and distributed infrastructures.
SoftBank and NVIDIA fast-forward AI-RAN commercialization
SoftBank has turned the AI-RAN vision into reality, with its successful outdoor field trial in Fujisawa City, Kanagawa, Japan, where NVIDIA-accelerated hardware and NVIDIA Aerial software served as the technical foundation.
This achievement marks multiple steps forward for AI-RAN commercialization and provides real proof points addressing industry requirements on technology feasibility, performance, and monetization:
- World’s first outdoor 5G AI-RAN field trial running on an NVIDIA-accelerated computing platform. This is an end-to-end solution based on a full-stack, virtual 5G RAN software integrated with 5G core.
- Carrier-grade virtual RAN performance achieved.
- AI and RAN multi-tenancy and orchestration achieved.
- Energy efficiency and economic benefits validated compared to existing benchmarks.
- A new solution to unlock AI marketplace integrated on an AI-RAN infrastructure.
- Real-world AI applications showcased, running on an AI-RAN network.
Above all, SoftBank aims to commercially release their own AI-RAN product for worldwide deployment in 2026.
To help other mobile network operators get started on their AI-RAN journey now, SoftBank is also planning to offer a reference kit comprising the hardware and software elements required to trial AI-RAN in a fast and easy way.
End-to-end AI-RAN solution and field results
SoftBank developed their AI-RAN solution by integrating hardware and software components from NVIDIA and ecosystem partners and hardening them to meet carrier-grade requirements. Together, the solution enables a full 5G vRAN stack that is 100% software-defined, running on NVIDIA GH200 (CPU+GPU), NVIDIA Bluefield-3 (NIC/DPU), and Spectrum-X for fronthaul and backhaul networking. It integrates with 20 radio units and a 5G core network and connects 100 mobile UEs.
The core software stack includes the following components:
- SoftBank-developed and optimized 5G RAN Layer 1 functions such as channel mapping, channel estimation, modulation, and forward-error-correction, using NVIDIA Aerial CUDA-Accelerated-RAN libraries
- Fujitsu software for Layer 2 functions
- Red Hat’s OpenShift Container Platform (OCP) as the container virtualization layer, enabling different types of applications to run on the same underlying GPU computing infrastructure
- A SoftBank-developed E2E AI and RAN orchestrator, to enable seamless provisioning of RAN and AI workloads based on demand and available capacity
The underlying hardware is the NVIDIA GH200 Grace Hopper Superchip, which can be used in various configurations from distributed to centralized RAN scenarios. This implementation uses multiple GH200 servers in a single rack, serving AI and RAN workloads concurrently, for an aggregated-RAN scenario. This is comparable to deploying multiple traditional RAN base stations.
In this pilot, each GH200 server was able to process 20 5G cells using 100-MHz bandwidth, when used in RAN-only mode. For each cell, 1.3 Gbps of peak downlink performance was achieved in ideal conditions, and 816Mbps was demonstrated with carrier-grade availability in the outdoor deployment.
AI-RAN multi-tenancy achieved
One of the first principles of AI-RAN technology is to be able to run RAN and AI workloads concurrently and without compromising carrier-grade performance. This multi-tenancy can be either in time or space: dividing the resources based on time of day or based on percentage of compute. This also implies the need for an orchestrator that can provision, de-provision, or shift workloads seamlessly based on available capacity.
At the Fujisawa City trial, concurrent AI and RAN processing was successfully demonstrated over GH200 based on static allocation of resources between RAN and AI workloads (Figure 1).
Each NVIDIA GH200 server constitutes multiple MIGs (Multi-Instance GPU), that enable a single GPU to be divided into multiple isolated GPU instances. Each instance has its own dedicated resources, such as memory, cache, and compute cores, and can operate independently.
The SoftBank orchestrator intelligently assigns whole GPUs or some MIGs within a GPU to run AI and some to run RAN workloads and switches them dynamically when needed. It is also possible to statically allocate a certain percentage of compute for RAN and AI, for example, 60% for RAN and 40% for AI instead of demand-based allocation.
The goal is to maximize capacity utilization. With AI-RAN, telcos can achieve almost 100% utilization compared to 33% capacity utilization for typical RAN-only networks. This is an increase of up to 3x while still catering to peak RAN loads, thanks to dynamic orchestration and prioritization policies.
Enabling an AI-RAN marketplace
With a new capacity for AI computing now available on distributed AI-RAN infrastructure, the question arises of how to bring AI demand to this AI computing supply.
To solve this, SoftBank used a serverless API powered by NVIDIA AI Enterprise to deploy and manage AI workloads on AI-RAN, with security, scale, and reliability. The NVIDIA AI Enterprise serverless API is hosted on the AI-RAN infrastructure and integrated with the SoftBank E2E AI-RAN orchestrator. It connects to any public or private cloud running the same API, to dispatch external AI inferencing jobs to the AI-RAN server when compute is available (Figure 2).
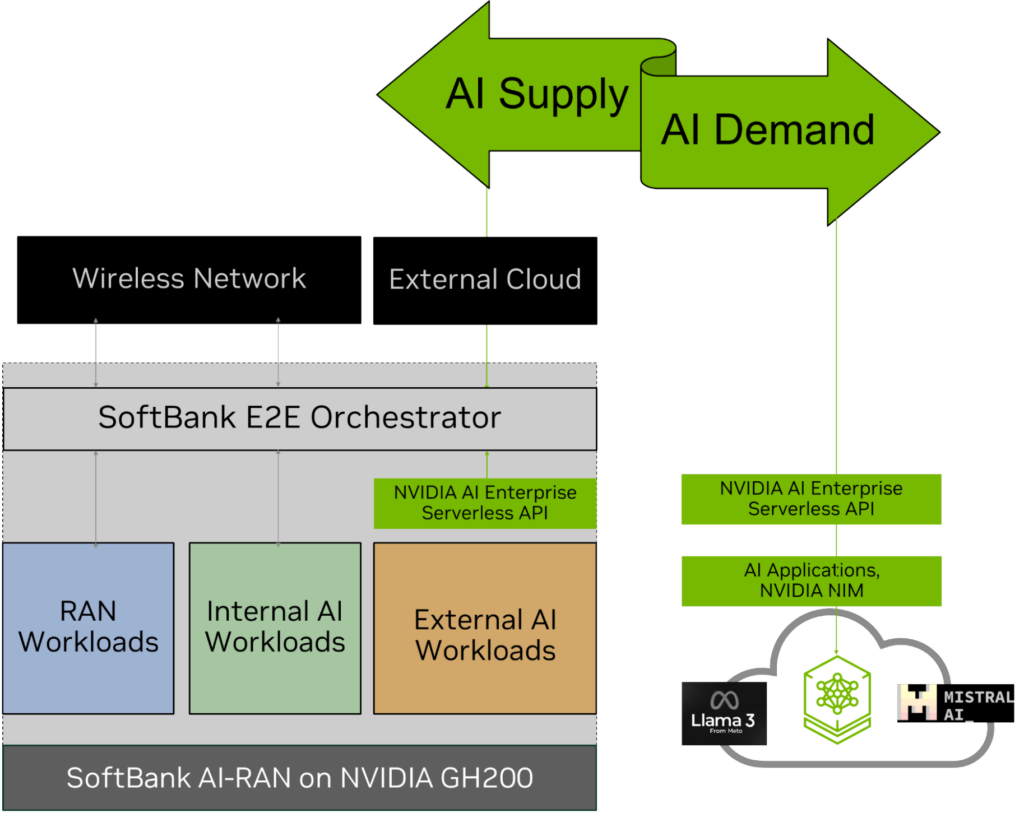
This solution enables an AI marketplace, helping SoftBank deliver localized, low-latency, secured inferencing services. It also demonstrated the importance of AI-RAN in helping telcos become the AI distribution grid, particularly for external AI inferencing jobs, and opened a new revenue opportunity.
AI-RAN applications showcased
In this outdoor trial, new edge AI applications developed by SoftBank were demonstrated over the live AI-RAN network:
- Remote support of autonomous vehicles over 5G
- Factory multi-modal AI applications
- Robotics applications
Remote support of autonomous vehicles over 5G
The key requirements of the social implementation of autonomous driving are vehicle safety and reducing operational costs.
At the Fujisawa City trial, SoftBank demonstrated an autonomous vehicle, relaying its front camera video using 5G to an AI-based remote support service hosted on the AI-RAN server. Multi-modal AI models analyzed the video stream, did risk assessment, and sent recommended actions to autonomous vehicles using text over 5G.
This is an example of explainable AI as well, as all the actions of the autonomous vehicle could be monitored and explained through summarized text and logging for remote support.
Factory multi-modal AI applications
In this use case, multi-modal inputs including video, audio, and sensor data, are streamed using 5G into the AI-RAN server. Multiple LLMs, VLMs, retrieval-augmented generation (RAG) pipelines, and NVIDIA NIM microservices hosted on the AI-RAN server are used to coalesce these inputs and make the knowledge accessible through a chat interface to users using 5G.
This fits well for factory monitoring, construction site inspections, and similar complex indoor and outdoor environments. The use case demonstrates how edge AI-RAN enables local data sovereignty by keeping data access and analysis local, secure, and private, which is a mandatory requirement of most enterprises.
Robotics applications
SoftBank demonstrated the benefit of edge AI inferencing for a robot connected over 5G. A robodog was trained to follow a human based on voice and motion.
The demo compared the response time of the robot when the AI inferencing was hosted on the local AI-RAN server to when it was hosted on the central cloud. The difference was apparent and obvious. The edge-based inference robodog followed the human’s movements instantly, while the cloud-based inference robot struggled to keep up.
Accelerating the AI-RAN business case with the Aerial RAN Computer-1
While the AI-RAN vision has been embraced by the industry, the energy efficiency and economics of GPU-enabled infrastructure remain key requirements, particularly how they compare to traditional CPU– and ASIC-based RAN systems.
With this live field trial of AI-RAN, SoftBank and NVIDIA have not only proven that GPU-enabled RAN systems are feasible and high-performant, but they are also significantly better in energy efficiency and economic profitability.
NVIDIA recently announced the Aerial RAN Computer-1 based on the next-generation NVIDIA Grace Blackwell superchips as the recommended AI-RAN deployment platform. The goal is to migrate SoftBank 5G vRAN software from NVIDIA GH200 to NVIDIA Aerial RAN Computer-1 based on GB200-NVL2, which is an easier shift given the code is already CUDA-ready.
With GB200-NVL2, the available compute for AI-RAN will increase by a factor of 2x. The AI processing capabilities will improve by 5x for Llama-3 inferencing, 18x for data processing, and 9x for vector database search compared to prior H100 GPU systems.
For this evaluation, we compared the target deployment platform, Aerial RAN Computer-1 based on GB200 NVL2, with the latest generation of x86 and the best-in-class custom RAN product benchmarks and validated the following findings:
- Accelerated AI-RAN offers best-in-class AI performance
- Accelerated AI-RAN is sustainable RAN
- Accelerated AI-RAN is highly profitable
Accelerated AI-RAN offers best-in-class AI performance
In 100% AI-only mode, each GB200-NVL2 server generates 25000 tokens/second, which translates to $20/hr of available monetizable compute per server, or $15K/month per server.
Keeping in mind that the average revenue per user (ARPU) of wireless services today ranges between $5–50/month depending on the country, AI-RAN opens a new multi-billion-dollar AI revenue opportunity that is orders of magnitude higher than revenues from RAN-only systems.
The token AI workload used is Llama-3-70B FP4, showcasing that AI-RAN is already capable of running the world’s most advanced LLM models.
Accelerated AI-RAN is sustainable RAN
In 100% RAN-only mode, GB200-NVL2 server power performance in Watt/Gbps shows the following benefits:
- 40% less power consumption than the best-in-class custom RAN-only systems today
- 60% less power consumption than x86-based vRAN
- Similar efficiencies across distributed-RAN and centralized-RAN configurations
For an even comparison, this assumes the same number of 100-MHz 4T4R cells and 100% RAN-only workload across all platforms.
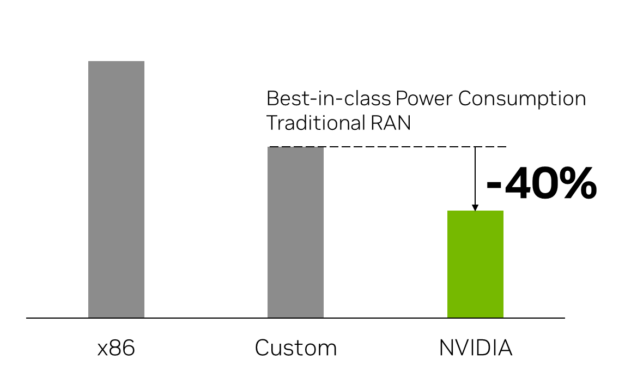
Accelerated AI-RAN is highly profitable
For this evaluation, we used the scenario of covering one district in Tokyo with 600 cells as the common baseline for RAN deployment for each of the three platforms being compared. We then looked at multiple scenarios for AI and RAN workload distribution, ranging from RAN-only to RAN-heavy or AI-heavy.
In the AI-heavy scenario (Figure 4), we used a one-third RAN and two-third AI workload distribution:
- For every dollar of CapEx investment in accelerated AI-RAN infrastructure based on NVIDIA GB200 NVL2, telcos can generate 5x the revenue over 5 years.
- From a net profit perspective, the overall investment delivers a 219% profit margin, considering all CapEx and OpEx costs.This is of course specific to SoftBank, as it uses local country costs assumptions.
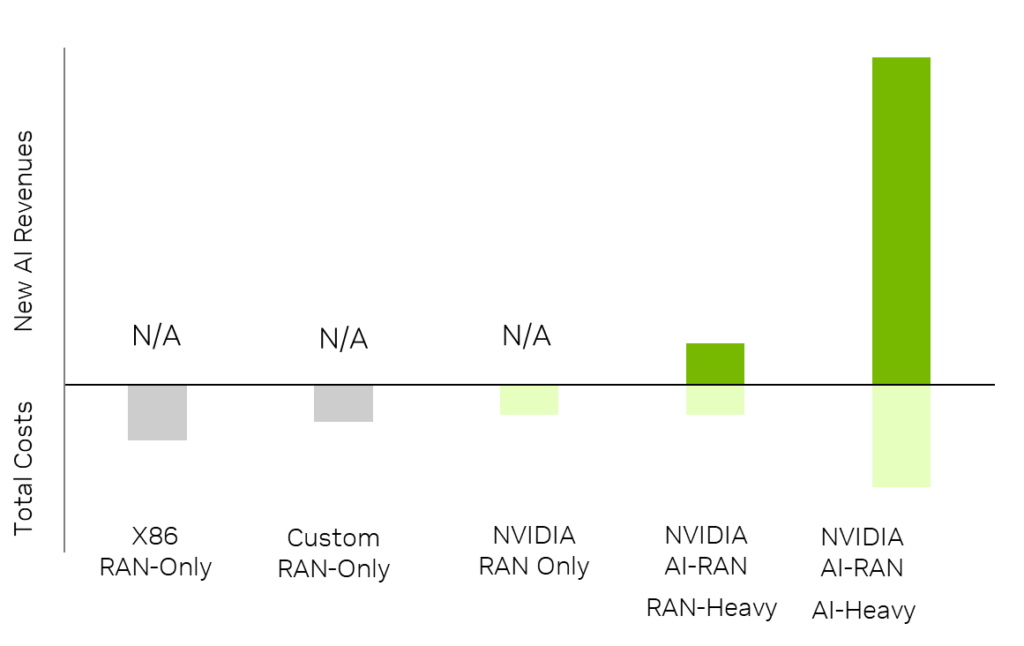
33% AI and 67% RAN | 67% AI and 33% RAN | |
$ of revenue per $ of CapEx | 2x | 5x |
Profit margin % based on TCO | 33% | 219% |
In the RAN-heavy scenario, we used two-thirds RAN and one-third AI workload distribution and found that revenue divided by CapEx for NVIDIA-accelerated AI-RAN is 2x, with a 33% profit margin over 5 years, using SoftBank local cost assumptions.
In the RAN-only scenario, NVIDIA Aerial RAN Computer-1 is more cost-efficient than custom RAN-only solutions, which underscores the benefits of using accelerated computing for radio signal processing.
From these scenarios, it is evident that AI-RAN is highly profitable as compared to RAN-only solutions, in both AI-heavy and RAN-heavy modes. In essence, AI-RAN transforms traditional RAN from a cost center to a profit center.
The profitability per server improves with higher AI use. Even in RAN-only, AI-RAN infrastructure is more cost-efficient than custom RAN-only options.
Key assumptions used for the revenue and TCO calculations include the following:
- The respective number of platforms, servers, and racks for each platform are calculated using a common baseline of deploying 600 cells on the same frequency, 4T4R.
- The total cost of ownership (TCO) is calculated over 5 years and includes the cost of hardware, software, and vRAN and AI operating costs.
- For the new AI revenue calculation, we used $20/hr/server based on GB200 NVL2 AI performance benchmarks.
- OpEx costs are based on local Japan power costs and aren’t extensible worldwide.
- Profit margin % = (new AI revenues – TCO) / TCO
This validation of AI revenue upside, energy efficiency, and profitability of AI-RAN leaves no doubts about the feasibility, performance, and economic benefits of the technology.
Going forward, exponential gains with each generation of NVIDIA superchips, such as Vera Rubin, will multiply these benefits by orders of magnitude further, enabling the much-awaited business transformation of telco networks.
Looking ahead
SoftBank and NVIDIA are continuing to collaborate toward the commercialization of AI-RAN and bringing new applications to life. The next phase of the engagements will entail work on AI-for-RAN to improve spectral efficiency and on NVIDIA Aerial Omniverse digital twins to simulate accurate physical networks in the digital world for fine-tuning and testing.
NVIDIA AI Aerial lays the foundation for operators and ecosystem partners globally to use the power of accelerated computing and software-defined RAN + AI to transform 5G and 6G networks. You can now use NVIDIA Aerial RAN Computer-1 and AI Aerial software libraries to develop your own implementation of AI-RAN.
NVIDIA AI Enterprise is also helping create new AI applications for telcos, hostable on AI-RAN, as is evident from this trial where many NVIDIA software toolkits have been used. This includes NIM microservices for generative AI, RAG, VLMs, NVIDIA Isaac for robotics training, NVIDIA NeMo, RAPIDS, NVIDIA Triton for inferencing, and a serverless API for AI brokering.
The telecom industry is at the forefront of a massive opportunity to become an AI service provider. AI-RAN can kickstart this new renaissance for telcos worldwide, using accelerated computing as the new foundation for wireless networks.
This announcement marks a breakthrough moment for AI-RAN technology, proving its feasibility, carrier-grade performance, superior energy efficiency, and economic value. Every dollar of CapEx invested in NVIDIA-accelerated AI-RAN infrastructure generates 5x revenues, while being 6G-ready.
The journey to AI monetization can start now.
AI-RAN Goes Live and Unlocks a New AI Opportunity for Telcos